What are the best ordinal scale examples? At its core, an ordinal scale categorizes data into distinct categories that are inherently ordered or ranked according to a specific criterion. They’re the bridge between the qualitative and the quantitative, allowing us to rank data based on some order without getting bogged down in the exact distance between those ranks.
Table of Contents
These scales find their use everywhere—from academic studies and market research to health evaluations and social surveys. By breaking down complex concepts into ordered categories, ordinal scale example helps us get a handle on preferences, attitudes, and performance levels.
Let’s dive into what makes ordinal scales tick, how they’re used across various fields, and the mix of strengths and challenges they bring to the table.
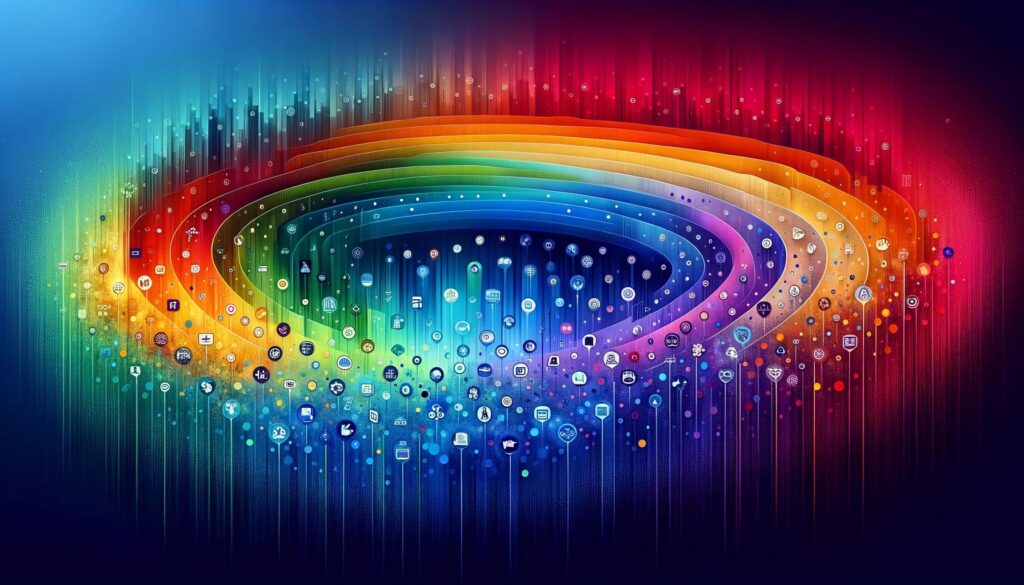
What is Ordinal Scale Example?
Ordinal scales are about order. They let us sort data into ranked categories based on a set hierarchy, setting them apart from nominal data, which is all about labeling without any order.
Unlike interval and ratio scales that tell us the exact difference between points, ordinal scales keep it vague. They’re perfect for when you can rank things but can’t (or don’t need to) measure the exact gap between those ranks.
The beauty of ordinal scales is in their simplicity and flexibility. They shine in situations where the order matters more than the precise numerical differences. Knowing their limitations, especially in statistical analysis, is key to making the most of the insights they offer.
Ordinal Scale Examples in Various Fields
Survey Responses and Market Research
In the world of market research, ordinal scales are invaluable. They help gauge consumer feelings and product feedback through tools like Likert scales (“Strongly Disagree” to “Strongly Agree”). This approach segments markets and spots trends in customer satisfaction, guiding strategic moves.
Education and Academic Research
The education field loves ordinal scales for ranking performances with letter grades (A-F) or sorting research findings by evidence levels. These scales organize data neatly, from class standings to journal rankings, offering a clear picture of academic merit and research impact.
Healthcare and Medicine
Ordinal scales in healthcare help gauge patient conditions, from pain levels to disease stages. They enable doctors to tailor treatments and prognoses without needing exact measurements, a crucial advantage in patient care.
Sports and Competitions
Sports competitions naturally align with ordinal scales, ranking athletes or teams by performance. This method is seen in Olympic medals, tennis rankings, or football leagues, where placement signifies relative success without detailing the performance gap.
Social Sciences and Psychology
In social sciences and psychology, ordinal scales measure attitudes, behaviors, and feelings. These tools capture data on inherently subjective matters, aiding the study of social trends, psychological states, and behavioral patterns.
Military Ranks
Military organizations use ordinal scales to delineate the hierarchy, from privates to generals. This structure orders personnel by their level of authority and responsibility, indicating their position in the chain of command without quantifying the extent of their power or experience.
Product Reviews
Star ratings for products or services are a classic ordinal scale application. Ranging from one (poor) to five stars (excellent), these ratings let users express their satisfaction level, though they don’t reveal the specifics behind their assessments.
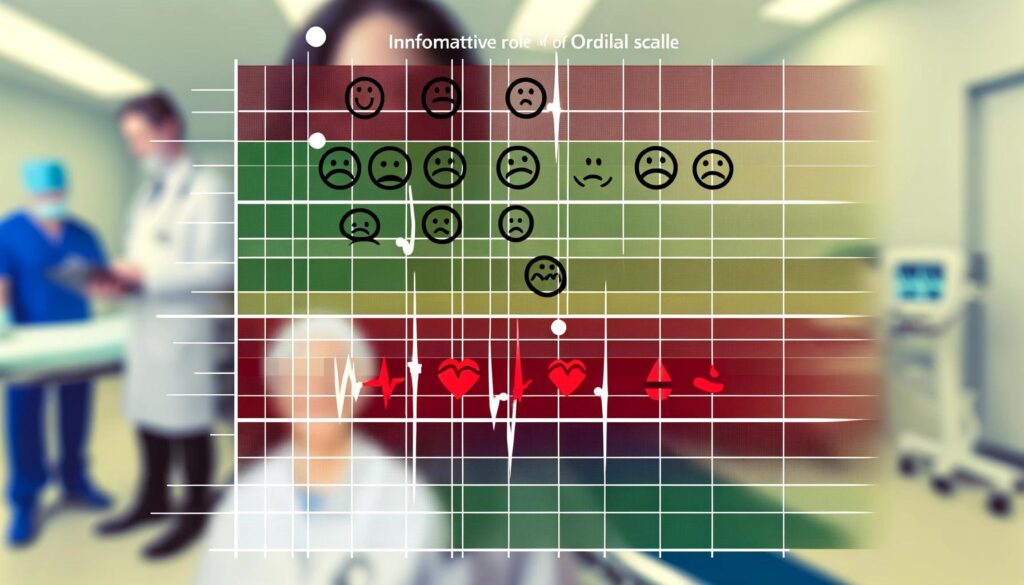
Analyzing Data with Ordinal Scales
Analyzing data that comes from ordinal scales is a bit like navigating without exact distances; you know the order of destinations, but not how far apart they are. This unique challenge requires a deep understanding of what ordinal data represents and which statistical tools are best suited for it. Unlike interval or ratio data, where each step is uniform and measurable, ordinal data sorts categories by order without indicating the size of the gap between them.
Grasping Ordinal Data
Ordinal data is all about ranking. It can show preferences or severity levels but stops short of quantifying the differences. This is what sets it apart from more quantifiable data types, demanding a tailored approach to analysis. For example, we can rank Olympic medals, but the exact performance gap between gold and silver remains undefined.
Statistical Tools for Ordinal Data
Given ordinal data’s qualitative nature, non-parametric statistics often come into play. These methods don’t presume data to follow a normal distribution, making them a better fit for ordinal’s often irregular spread.
- Mann-Whitney U Test: Useful for comparing two unrelated groups on an ordinal scale to see if there’s a notable difference in their median ranks.
- Kruskal-Wallis H Test: An advanced version of the Mann-Whitney test, this is used when comparing more than two groups to identify any significant ranking disparities.
- Spearman’s Rank Correlation Coefficient: This evaluates the relationship between two variables, ideal for assessing the association strength and direction in ordinal data sets.
- Ordinal Logistic Regression: Tailored for situations where the outcome is ordinal, this method helps model the relationship between that outcome and one or more predictor variables.
Navigating the Challenges
A common pitfall is applying parametric methods, suited for interval or ratio data, to ordinal scales. This can lead to inaccurate analyses because it overlooks the non-quantitative nature of ordinal intervals. Additionally, interpreting results demands an acknowledgment of these limits, as significant differences found in tests might not clarify the extent of these differences.
Best Practices
For robust analysis, it’s crucial to:
- Select non-parametric tests that align with your data and research questions.
- Resist treating ordinal data as interval or ratio without solid reasoning.
- Communicate the constraints of ordinal data and your analytical approach when sharing findings.
Advantages, Limitations and Considerations
While ordinal scales are a staple across numerous fields for their simplicity and versatility, navigating their benefits and drawbacks is essential for effective use.
Advantages of Ordinal Scales
- Ease of Creation and Interpretation: Their straightforward nature makes ordinal scales user-friendly for both researchers and participants, ideal for capturing complex phenomena that are hard to quantify.
- Adaptable Across Disciplines: Their flexibility allows for application in various contexts, from healthcare assessments to consumer preference surveys, providing valuable insights into rankings and degrees of agreement.
- Depth of Qualitative Data: Ordinal scales excel in revealing patterns in attitudes and behaviors that might be lost in purely nominal data, enriching our understanding of preferences and perceptions.
Limitations of Ordinal Scales
- Quantitative Limits: The inability to measure the exact difference between ranks curtails the depth of statistical analysis possible, potentially glossing over variations within the data.
- Risks of Misinterpretation: Without clear communication, the meaning behind ordinal rankings can be misunderstood, leading to incorrect assumptions about the equality of differences across ranks.
- Subjective Category Definitions: The creation and interpretation of scale points can introduce bias, especially if the ranking criteria are vague or interpreted variably by respondents.
Making the Most of Ordinal Scales
Effective application hinges on:
- Defining scale points clearly to ensure consistent interpretation.
- Employing statistical methods that respect the data’s ordinal nature to avoid misleading conclusions.
- Being mindful of response distribution across categories, as imbalances can affect statistical reliability and interpretive clarity.
- Reporting methods and findings transparently, emphasizing the data’s ordinal nature and the rationale behind analytical choices.
Takeaways
Above the perfect ordinal scale examples you could use to understand the concept! Ordinal scales are an essential tool for researchers, shedding light on diverse phenomena across fields. Their careful use and analysis can reveal patterns and trends vital for informed decisions, policy crafting, and scientific progress. Grasping the subtleties of ordinal scales is fundamental for anyone eager to navigate the complexities of data in pursuit of significant insights.
Understanding Ordinal Scales
In sum, while ordinal scales offer a powerful means to rank and categorize data, their fruitful use demands careful planning, appropriate analysis, and clear communication. By acknowledging their strengths and limitations, researchers can harness ordinal scales to uncover meaningful insights into various phenomena.
Boosting creativity with Internet apps? Follow aneasytool.com for inspiration and guidance.